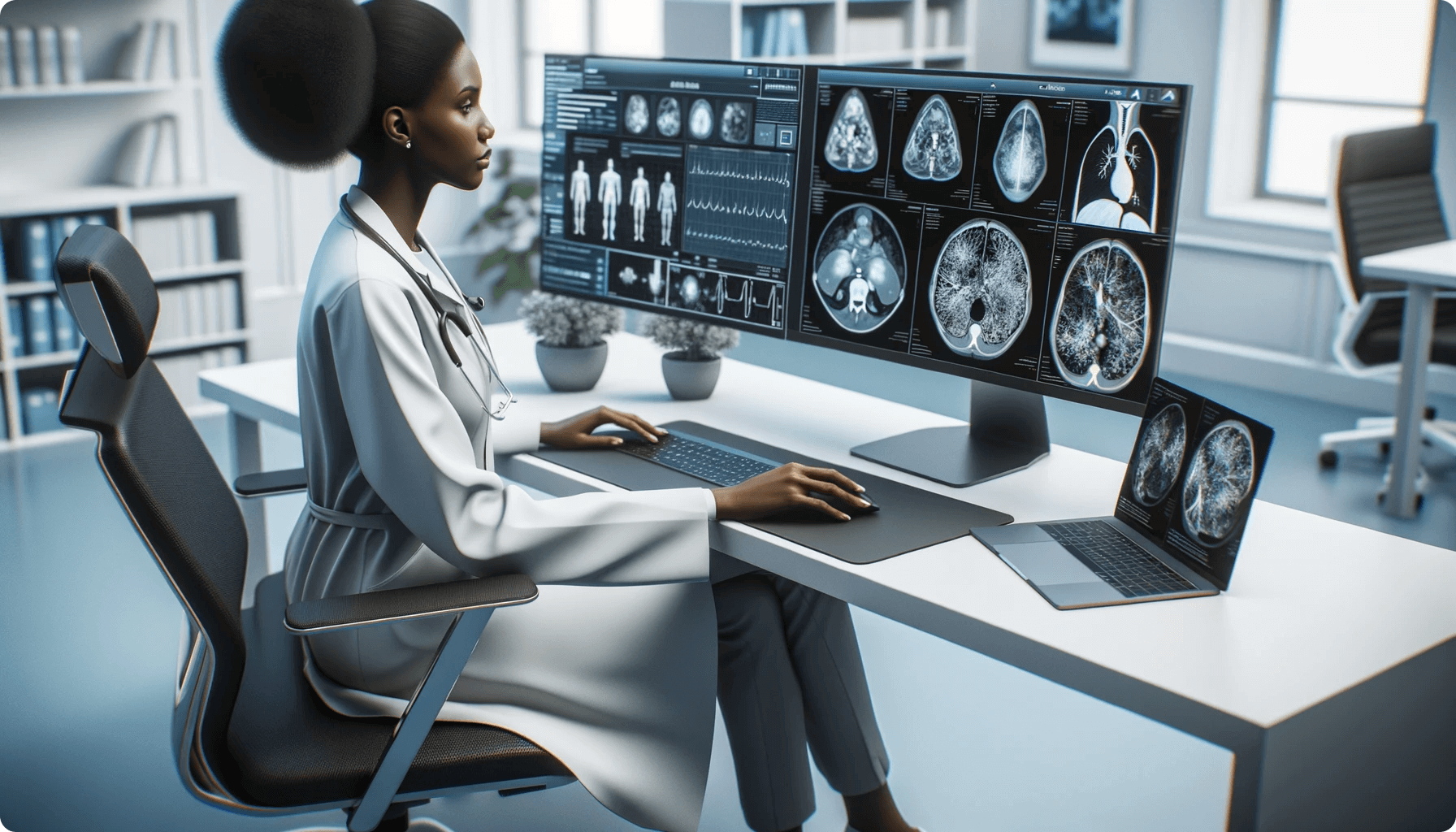
We had the opportunity earlier this Spring to share our technical perspectives as input into the development of the National Artificial Intelligence Research and Development Strategic Plan being developed with the leadership of the White House Office of Science and Technology Policy (OSTP). Here is what we shared in our letter and we always welcome the thoughts of our broader community as well!
Q Bio recognizes that Artificial Intelligence (AI) and Machine Learning (ML) technologies have the potential to transform health care by deriving new and important insights from the vast amount of data generated in the industry. Medical devices are using these technologies to innovate their products to better assist health care providers and improve patient care. There has been early adoption of AI / ML in the medical imaging industry and with the early adoption, there have been questions raised around guidance of use. As a leader in health technology and in the space of imaging and medical bioinformatics, Q Bio believes the following principles around AI adoption and use should be considered.
1. Most techniques labeled as AI / ML at this time are in essence statistical algorithms that learn from a large set of consistent training data and can make inferences from new data that has very similar properties as the training data. The benefit of this approach is identifying correlations in the data that are not easily described by humans and lead to very powerful applications with promising performance in many applications. However, most of these techniques do not “understand” the data. They operate on correlation but not causation. There should be caution around application as they can create data by interpolating from past training data. In the academic world, there has been significant progress in recent years on techniques allowing causal inference and associated network techniques, which if supported for further development could mean a leap forward in AI, i.e. networks would not only be able to detect correlations between smoking and lung cancer, but actually be able to infer that smoking causes cancer. We believe further research in this domain will have an impact on public health management and disease discovery.
2. We are concerned with the overuse and perhaps misappropriation of neural networks in medical imaging applications. In many cases, networks are being used to provide de-noised, resolution-increased, or even image contrast transferred data (i.e. a CT image generated from MRI data by a neural network) for diagnosis. While there is a certain “wow factor” with these applications, there is a lack of awareness that these networks are simply interpolating data, or in some cases restoring data using correlations from the training dataset. In other words, while these applications may help display images in a form more familiar to a physician, they don’t actually enhance data, or show smaller structures in super-resolution images, as the information is not contained in the original data. This can be very powerful, but the output is non-deterministic and not easy to audit.
3. We also observe in our industry that networks have been used to supplant algorithms that are analytical and verifiable, often by the laws of physics or mathematics, resulting in a new application that is no longer as easy to verify as the analytical solution. Clear guidelines should be in place around the communication of the limitations and risks associated with the use of neural networks, especially outside single-purpose applications. While the FDA is implementing stricter regulations around the verification of such applications, we believe that even the R&D world should be focused on the appropriate use of neural networks, rather than a wide range of transitions to neural networks, where they are not necessary or even more harmful.
4. We believe a good use of AI and neural networks and other machine learning techniques is in the acceleration of conventional algorithms. For example, in the area of optimization, techniques such as auto-differentiation have made an impact by enabling minimization of cost functions that only few trained specialists were able to address in the past. By embedding ML into conventional algorithms, verification of the solution and detection of failure is much easier to ensure.
5. Neural networks are safest to use when we may not be able to compute an answer we want directly from raw inputs, but can verify correctness of the neural network output efficiently. An example of this is protein folding. It is computationally intractable to directly compute how a protein will fold. If we train a neural network to predict how it will fold, we can use the laws of physics to then verify that the output of the neural network is at least a valid solution because it does not break those laws. On the other hand, neural networks are most dangerous to use where it is not possible to directly compute answers over inputs, and it is not tractable to verify correctness of the answer given by algorithm.
At Q Bio we believe neural networks are a very useful tool, but for the foreseeable future they will be tools that help clinicians make better and more efficient decisions and will not be a replacement for human decision making. Neural networks will just supply another input that clinicians can use in their practice. We will continue to focus on using neural networks on problems where we can verify the accuracy of the output of the neural network easily, or where we are confident we can train neural networks on data that uniformly samples entire input spaces, not subspaces. Overall, we continue to believe in the power of AI/ML and with responsible application of this technology, we believe the U.S. will continue to be a leader in innovation. We strongly support ongoing investment in Artificial Intelligence and Machine Learning Research and public private partnerships. We at Q Bio will continue to invest in our applications of AI/ML in bringing cheaper, faster, better and quantified imaging to all.